Graphical abstract
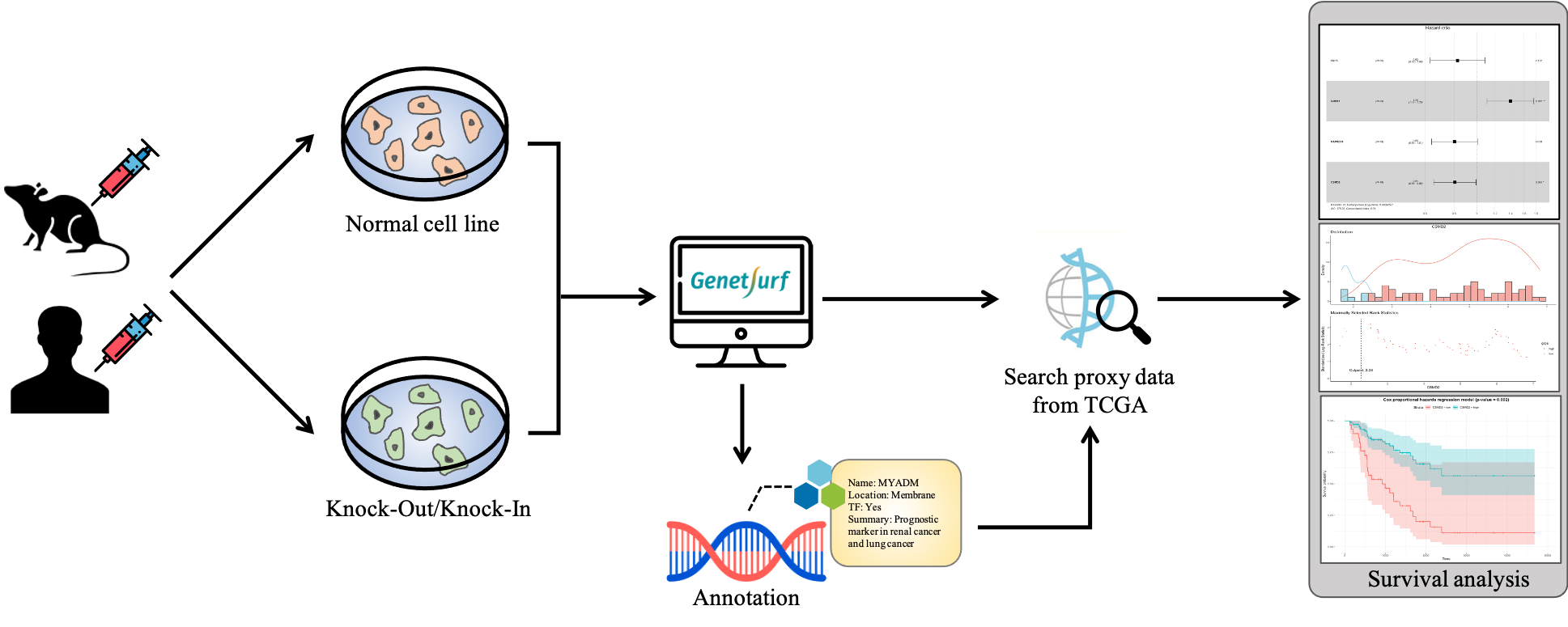
The digital visualization tool for Survival analysis with the Gene Network viewer
Like Genet, GenetSurf is the easy, agile way to rapidly surf through open-source datasets.
Our platform, GenetSurf, is a useful bioinformatic tool for identifying gene-sets which potentially affect patient survival outcomes. This tool only takes one input file and expression profile (e.g., gene expression of a cell line array). The user can select their target genes before performing the analysis. The most powerful function in the tool is inferring the relationship between survival outcome and gene-set without a self-provided patient survival. The idea behind this is that we link open-source expression profiles with the self-provided expression profile by gene network similarity. Our platform may help users imagine how the genes they select will change the patient's survival outcome and design the wet-lab experiment based on the result.
Gene networks provide a gene-gene interaction and give us basic insight into biological processes. Recently, many researches consider gene-sets or pathways instead of a single gene to model the relationship between phenotype and genotype. Thus, our platform illustrates the gene network related to target genes or differentially expressed genes before performing survival analysis. The undirected gene network, which is inferred by different network inference methods, is most often used in the research. However, we plan to use Bayesian graphical model to construct the directed gene network to give a comprehensive perspective of biological processes.
In addition, we propose a novel idea to link open-source expression profiles with the self-provided expression profile. We calculate the gene network similarity between the self-provided expression profile and more than 30 open-source expression profiles from The Cancer Genome Atlas (TCGA) and report the open-source expression profile with the highest network similarity (suggest similarity > 80%) as the proxy expression profile. The open-source data we use contains both gene expression and survival information, thus it allows us to do the survival analysis. The result of survival analysis is beneficial for users because the proxy expression profile and self-provided expression profile are similar enough based on the network similarity. Moreover, the network comparison method for network similarity is the euclidean distance method which is a kind of Known Node-Correspondence (KNC) method.
For users with biological backgrounds, our platform also provides the summary description of each gene from the gene network viewer. The basic information includes transcription factor genes, relationships between genes and the subcellular location parsed from The Human Protein Atlas. It is only the first step to integrate the external knowledgebase. In this project, we will integrate validated network databases such as the KEGG pathway database into the survival model.
To use GenetSurf, please contact Taiwan AILabs.